AI models have made significant strides in generating essays and various types of text. However, solving math problems, which require logical reasoning, remains a challenge for most current AI systems.
That might be changing. Google DeepMind has announced that it has trained two specialized AI systems to tackle complex math problems that demand advanced reasoning. These systems, named AlphaProof and AlphaGeometry 2, collaborated to solve four out of six problems from this year’s International Mathematical Olympiad, a prestigious competition for high school students. This achievement is equivalent to winning a silver medal.
This marks the first time any AI system has achieved such a high success rate on these types of problems. “This is great progress in the field of machine learning and AI,” says Pushmeet Kohli, vice president of research at Google DeepMind. “No such system has been developed until now which could solve problems at this success rate with this level of generality.”
Math problems that involve advanced reasoning are particularly challenging for AI systems due to their need for forming and drawing on abstractions, complex hierarchical planning, setting subgoals, backtracking, and exploring new paths.
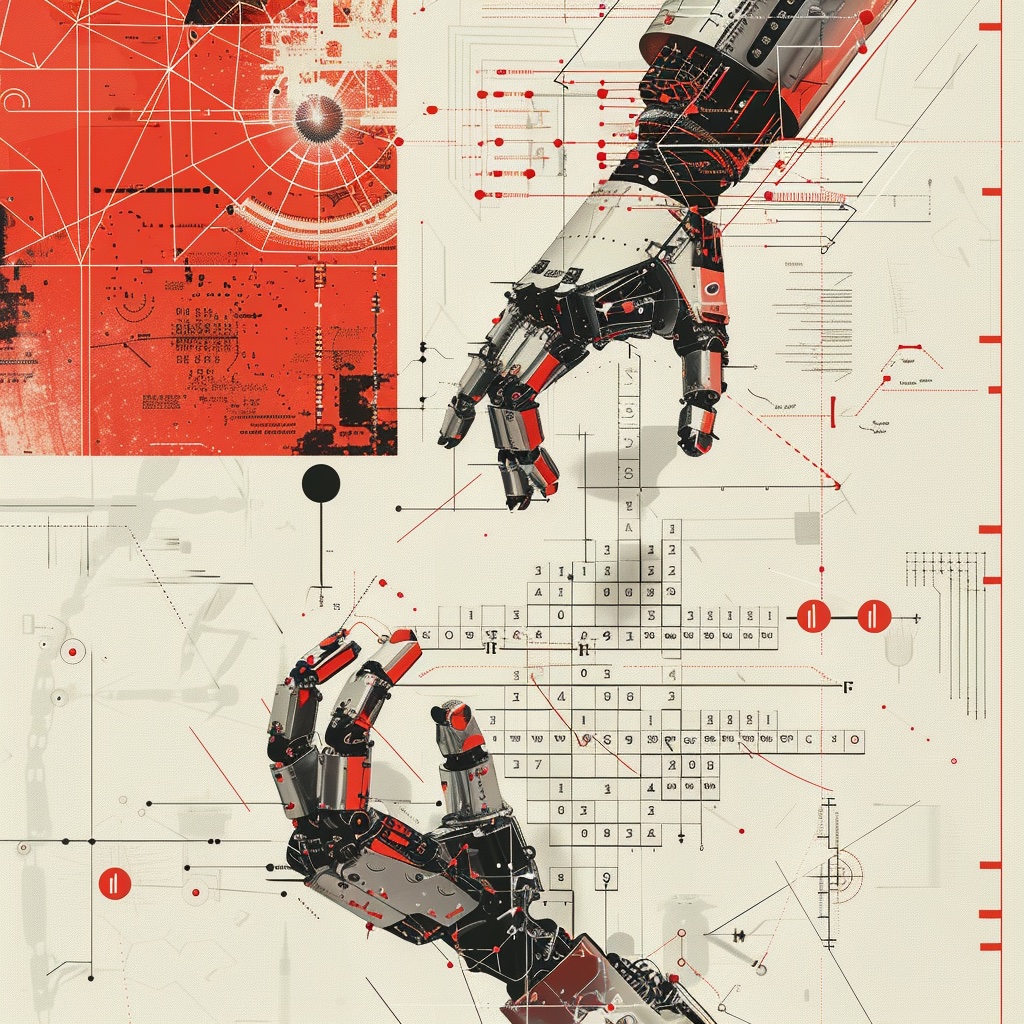
“It is often easier to train a model for mathematics if you have a way to check its answers (e.g., in a formal language), but there is comparatively less formal mathematics data online compared to free-form natural language (informal language),” says Katie Collins, a researcher at the University of Cambridge specializing in math and AI, who was not involved in the project.
Google DeepMind aimed to bridge this gap by creating AlphaProof, a reinforcement-learning-based system trained to prove mathematical statements in the formal programming language Lean. A key component is a version of DeepMind’s Gemini AI, fine-tuned to translate math problems phrased in natural, informal language into formal statements. This created a large library of formal math problems with varying degrees of difficulty.
Automating the translation of data into formal language is a significant advancement for the math community, according to Wenda Li, a lecturer in hybrid AI at the University of Edinburgh, who peer-reviewed the research but was not involved in the project. “We can have much greater confidence in the correctness of published results if they are able to formulate this proving system, and it can also become more collaborative,” he says.
The Gemini model collaborates with AlphaZero, a reinforcement-learning model trained to master games like Go and chess, to prove or disprove millions of mathematical problems. The more problems AlphaProof solves, the better it becomes at handling increasingly complex issues.
While AlphaProof was designed to address a wide range of mathematical topics, AlphaGeometry 2—an enhanced version of a system announced by Google DeepMind in January—focuses on problems involving movements of objects and equations related to angles, ratios, and distances. It was trained on significantly more synthetic data than its predecessor, enabling it to tackle more challenging geometry questions.
To evaluate the systems, Google DeepMind researchers assigned them the six problems presented to human competitors in this year’s IMO and tasked them with proving the correctness of their answers. AlphaProof solved two algebra problems and one number theory problem, including the competition’s most difficult one. AlphaGeometry 2 successfully solved a geometry question, but two combinatorics questions remained unsolved.
“Generally, AlphaProof performs much better on algebra and number theory than combinatorics,” says Alex Davies, a research engineer on the AlphaProof team. “We are still working to understand why this is, which will hopefully lead us to improve the system.”
The systems’ submissions were evaluated by renowned mathematicians Tim Gowers and Joseph Myers, who awarded full marks for each of their four correct answers, totaling 28 out of a possible 42 points. A human competitor achieving this score would receive a silver medal, just missing the gold threshold of 29 points.
This accomplishment is the first time any AI system has reached a medal-level performance on IMO questions. “As a mathematician, I find it very impressive, and a significant jump from what was previously possible,” Gowers said during a press conference.
Myers concurred, noting the substantial advancement over previous AI capabilities. “It will be interesting to see how things scale and whether they can be made faster, and whether it can extend to other sorts of mathematics,” he said.
According to Collins, creating AI systems capable of solving more challenging math problems could foster exciting human-AI collaborations, aiding mathematicians in solving and inventing new problems, and enhancing our understanding of human problem-solving in mathematics. “There is still much we don’t know about how humans solve complex mathematics problems,” she says.