While executives and managers are enthusiastic about integrating generative artificial intelligence and large language models into their operations, it’s essential to consider how these technologies will deliver business value. This area remains complex and requires new approaches and skillsets distinct from previous technology waves.
The challenge lies in monetizing AI’s impressive proofs of concept. Steve Jones, executive VP at IT company Capgemini, highlighted this issue at the recent Databricks conference in San Francisco. “Proving the ROI is the biggest challenge of putting 20, 30, 40 GenAI solutions into production,” he said.
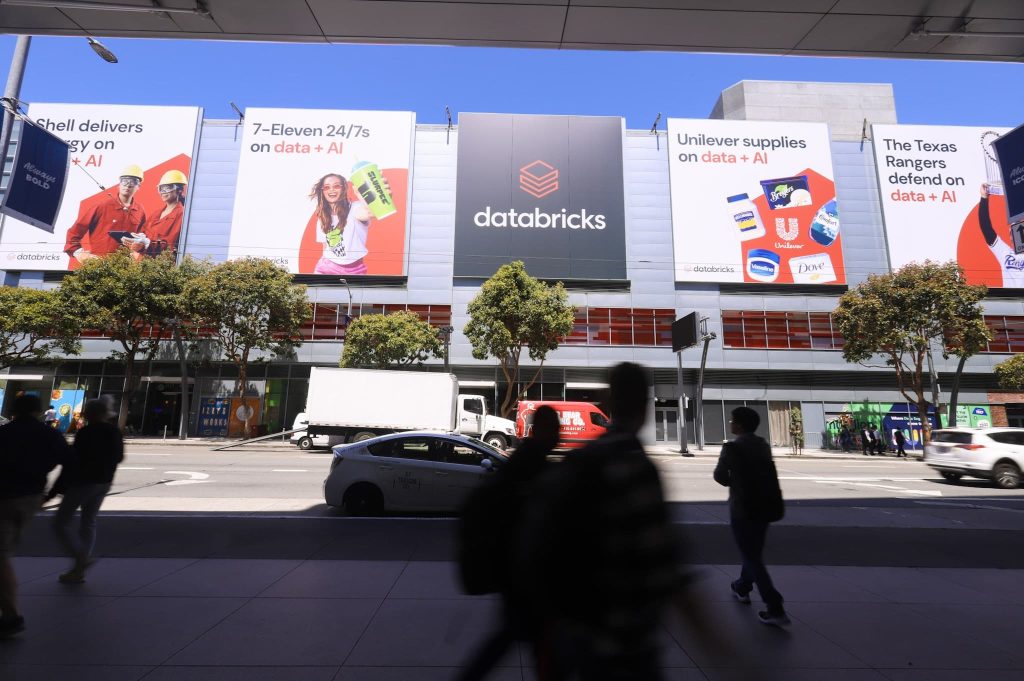
Investments in testing and monitoring LLMs are crucial to ensure accuracy and reliability. “You want to be a little bit evil to test these models,” Jones advised. Developers and QA experts should intentionally introduce errors during testing to evaluate the models’ resilience. Jones shared an example where he tricked a model by claiming a company was “using dragons for long-distance haulage.” The model responded with guidance on handling dragons and even suggested fire and safety training.
Jones emphasized that generative AI can easily be misused in applications, adding superficial features without proper security measures. “Gen AI is a phenomenal technology to just add some bells and whistles to an application, but truly terrible from a security and risk perspective in production,” he noted.
Generative AI is expected to reach mainstream adoption within two to five years, a rapid pace compared to other technologies. “Your challenge is going to be how to keep up,” said Jones. He described two scenarios: an overly optimistic vision of a single, flawless model and the reality of fierce competition among vendors and platforms, requiring robust infrastructure and guardrails.
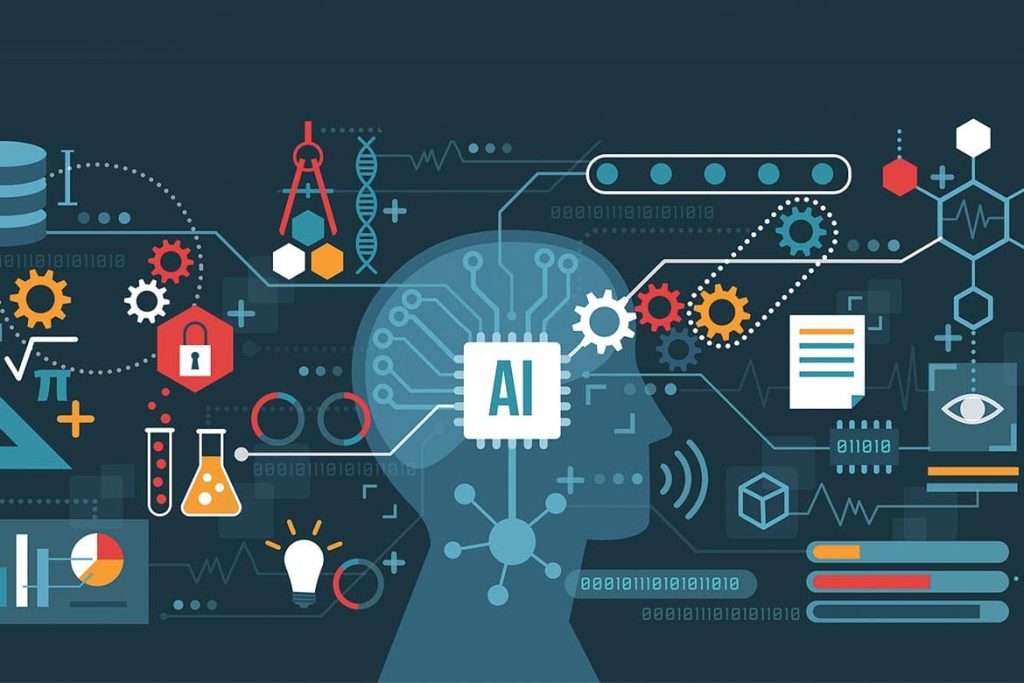
Applying LLMs to tasks that require less power, such as address matching, is another risk. “If you’re using one big model for everything, you’re basically just burning money,” Jones explained. He compared it to hiring a lawyer to write a birthday card at high rates. Vigilance for cost-effective uses of LLMs is key. “If something goes wrong, you need to be able to decommission a solution as fast as you can commission a solution,” he urged.
Jones recommended using multiple models to measure performance and response quality. “You should have a common way to capture all the metrics, to replay queries, against different models,” he said. Comparing models like GPT-4 Turbo and Llama helps find cost-effective solutions. “Because these models are constantly updating,” Jones added.